Artificial Intelligence (AI) has become an integral part of our lives, transforming the way we work, communicate, and make decisions. Within the realm of AI, two prominent approaches have emerged – Generative AI and Predictive AI. Both have their unique characteristics and applications.
In this article, we will delve deep into the intricacies of Generative AI and Predictive AI, exploring their differences, advantages, disadvantages, and the roles they play in today’s fast-paced world. So let’s dive in!
Understanding the Basics of AI
Before we delve into the specifics of Generative AI and Predictive AI, it’s crucial to have a solid foundation in the basics of AI. AI refers to the simulation of human intelligence in machines that are programmed to think, reason, and learn. It encompasses various techniques and methodologies that enable machines to perform tasks that typically require human cognitive abilities.
Artificial intelligence can be broadly classified into two categories – Generative AI and Predictive AI. Let’s explore each of these approaches in detail.
AI has seen significant advancements in recent years, with the development of sophisticated algorithms and computing power. These advancements have enabled AI systems to process vast amounts of data and make complex decisions in real-time. As a result, AI has been integrated into various aspects of our daily lives, from virtual assistants on our smartphones to self-driving cars on the roads.
Furthermore, AI is not limited to just one approach or technique. It encompasses a wide range of subfields, including machine learning, natural language processing, computer vision, and robotics. Each subfield focuses on different aspects of AI, such as pattern recognition, language understanding, image analysis, and autonomous control.
What is Generative AI?
Generative AI, as the name suggests, focuses on generating new content or data based on existing information or patterns. It is designed to understand and replicate the creative processes of human beings. Generative AI models are trained on massive datasets and can produce new and original content, such as images, text, music, and even human-like conversations.
Generative AI has applications in various industries, including art, design, and entertainment. For example, artists and designers use generative AI tools to create unique and innovative designs, while musicians leverage generative AI to compose new music compositions. In the field of healthcare, generative AI is used to generate synthetic data for training medical imaging models, improving diagnostic accuracy and patient outcomes.
What is Predictive AI?
On the other hand, Predictive AI focuses on making predictions or forecasts based on historical and real-time data. It analyzes patterns, trends, and correlations in the data to predict future outcomes or behaviors. Predictive AI is widely used in various industries, including finance, healthcare, and marketing, to make informed decisions and gain valuable insights.
In finance, predictive AI models are used to forecast stock prices, detect fraudulent activities, and optimize trading strategies. In healthcare, predictive AI is employed to predict disease outbreaks, personalize treatment plans, and improve patient care. In marketing, predictive AI helps businesses analyze customer behavior, predict market trends, and optimize advertising campaigns for better results.
The Core Differences Between Generative and Predictive AI
While both Generative AI and Predictive AI fall under the umbrella of AI, they have distinct differences that set them apart. Let’s explore some of these differences:
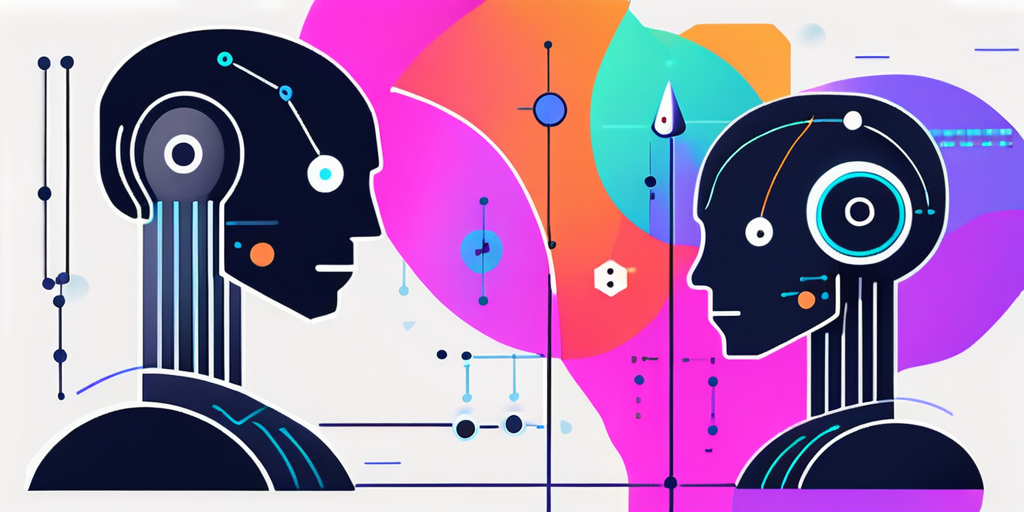
Generative AI, also known as creative AI, is a branch of artificial intelligence that focuses on creating new content, such as images, music, or text. It operates by learning patterns from a dataset and then generating new content based on that learning. This technology is often used in creative fields like art and music composition, where the goal is to produce original and innovative outputs.
On the other hand, Predictive AI is more concerned with making predictions or forecasts based on historical and real-time data. This type of AI is commonly used in applications like weather forecasting, stock market analysis, and customer behavior prediction. Predictive AI algorithms analyze patterns in data to make informed predictions about future events or trends.
Difference in Functionality
Generative AI focuses on creating new content, while Predictive AI is primarily concerned with making predictions or forecasts. Generative AI is more exploratory in nature, aiming to generate novel outputs, whereas Predictive AI aims to provide accurate predictions based on historical data.
Difference in Data Handling
Generative AI relies heavily on large, diverse datasets to learn and generate new content. It analyzes patterns and learns from the data to create something new. In contrast, Predictive AI relies on historical and real-time data to identify trends and patterns, enabling it to make accurate predictions.
Difference in Learning Approach
The learning approach of Generative AI and Predictive AI also differs. Generative AI models are trained through unsupervised learning, as they do not have specific target outputs to learn from. In contrast, Predictive AI models are trained through supervised or semi-supervised learning, as they are provided with labeled data to make future predictions.
In summary:
Feature | Generative AI | Predictive AI |
---|---|---|
Purpose | Designed to generate new content or data based on the training data it has been fed. | Designed to predict outcomes based on input data, often looking for patterns or trends that can forecast future events. |
Output | Can create entirely new content that didn’t exist before (e.g., images, text, music). | Provides predictions, classifications, or recommendations based on historical data. |
Techniques | Uses models like Generative Adversarial Networks (GANs), Variational Autoencoders (VAEs), and Transformer models for tasks like text generation. | Uses statistical models, machine learning models like regression, decision trees, neural networks, and time series forecasting models. |
Use Cases | Content creation, data augmentation, simulation, and creative applications in art, design, and entertainment. | Risk assessment, demand forecasting, spam detection, user behavior prediction, and financial market analysis. |
Data Handling | Learns the distribution of training data to generate new instances that are similar but not identical. | Analyzes patterns and relationships in historical data to make predictions about new data. |
Interactivity | In some applications, it can interact with users to refine outputs based on feedback (e.g., adjusting parameters for generation). | Typically, it involves less direct interaction with the user, focusing instead on analyzing input data to provide accurate predictions. |
Examples | Deepfake videos, AI-generated artwork, synthetic datasets for training other AI models. | Weather forecasting, stock market predictions, personalized recommendations in e-commerce platforms. |
The Pros and Cons of Generative AI

Generative AI offers several advantages that make it a valuable approach in certain domains:
Advantages of Generative AI
- Ability to create original content: Generative AI models can create new and unique content, enabling creativity and innovation in various fields.
- Enhanced data analysis: Generative AI can analyze complex datasets, extracting meaningful insights and patterns that humans may not easily identify.
- Diverse applications: Generative AI finds applications in image synthesis, text generation, music composition, and even virtual reality.
One of the most fascinating aspects of generative AI is its ability to mimic human creativity. By leveraging neural networks and machine learning algorithms, generative AI models can produce art, music, and literature that is indistinguishable from human-created content. This opens up new avenues for collaboration between humans and machines, where AI can assist artists, writers, and musicians in their creative processes.
Furthermore, generative AI has shown promising results in the field of healthcare. Researchers are exploring how AI-generated models can be used to simulate biological processes, predict disease outcomes, and even discover new drugs. By leveraging the power of generative AI, scientists can accelerate the pace of medical research and potentially revolutionize the healthcare industry.
While Generative AI offers exciting possibilities, it also comes with certain limitations:
Disadvantages of Generative AI
- Quality control challenges: Generating high-quality content consistently remains a challenge for Generative AI models.
- Ethical considerations: The potential misuse of Generative AI for creating deepfakes or spreading misinformation raises ethical concerns.
- Computational complexity: Training and running Generative AI models often require substantial computational resources.
Despite its potential benefits, the use of generative AI raises concerns about data privacy and security. As AI systems generate content based on vast amounts of data, there is a risk of unintentionally revealing sensitive information or perpetuating biases present in the training data. Safeguarding data integrity and ensuring that generative AI models adhere to ethical standards are crucial challenges that researchers and developers must address.
The Pros and Cons of Predictive AI
Like Generative AI, Predictive AI also brings several advantages and disadvantages to the table:
When delving into the advantages of Predictive AI, one cannot overlook the profound impact it has on businesses. One key advantage is its ability to provide accurate predictions. By analyzing vast amounts of historical data, Predictive AI can generate forecasts that help businesses make strategic and well-informed decisions. This predictive power is instrumental in anticipating market trends, customer behavior, and resource allocation.
Furthermore, Predictive AI contributes to improved efficiency within organizations. By automating predictive processes, AI eliminates the need for manual intervention, saving time, effort, and resources. This streamlined approach not only boosts productivity but also allows businesses to reallocate human capital to more creative and strategic tasks, ultimately enhancing overall operational efficiency.
Advantages of Predictive AI
- Accurate predictions: Predictive AI leverages historical data to make accurate forecasts and predictions, helping businesses make informed decisions.
- Improved efficiency: By automating predictive processes, AI can save time, effort, and resources compared to traditional manual approaches.
- Personalized recommendations: Predictive AI enables personalized recommendations in e-commerce, entertainment, and other industries, enhancing customer experiences.
Despite its advantages, Predictive AI faces certain challenges:
On the flip side, Predictive AI encounters obstacles that warrant attention. One significant challenge is its reliance on data. Predictive AI is heavily dependent on the availability of quality and relevant data. Inaccurate, incomplete, or biased data can significantly impact the accuracy and reliability of the predictions generated, leading to flawed insights and decision-making.
Moreover, the widespread adoption of Predictive AI raises valid concerns surrounding privacy and data protection. As AI systems collect and analyze vast amounts of sensitive information, questions regarding data security, user consent, and ethical use come to the forefront. Safeguarding personal data and ensuring compliance with data privacy regulations are crucial considerations in the deployment of Predictive AI technologies.
Disadvantages of Predictive AI
- Data dependency: Predictive AI heavily relies on the availability of quality and relevant data. Insufficient or biased data can impact the accuracy of predictions.
- Privacy concerns: The collection and use of sensitive data raise concerns regarding privacy and data protection.
- Black box problem: The inner workings of predictive AI models can sometimes be challenging to interpret, resulting in a lack of transparency.
Generative AI, also known as creative AI, is revolutionizing the way we approach creativity and innovation. Beyond just aiding in the creation of visual effects and digital imagery, Generative AI is being used to generate music, write poetry, and even design fashion. This technology is pushing the boundaries of what is possible in the creative realm, blurring the lines between human and machine-generated art.
Moreover, Generative AI is making waves in the field of healthcare by assisting in drug discovery, personalized medicine, and medical image analysis. By analyzing vast amounts of data and identifying patterns that may go unnoticed by human researchers, Generative AI is helping to accelerate the pace of medical advancements and improve patient outcomes.
The potential for Generative AI to revolutionize healthcare is immense, with researchers exploring applications in disease diagnosis, treatment optimization, and even surgical procedures.
The Role of Predictive AI in Today’s World
Predictive AI plays a crucial role in numerous industries, revolutionizing the way businesses operate. In finance, Predictive AI models help detect fraud, assess risks, and make data-driven investment decisions. In healthcare, Predictive AI assists in diagnosing diseases, predicting patient outcomes, and optimizing treatment plans. Moreover, Predictive AI has proven beneficial in marketing by enabling targeted advertising, customer segmentation, and demand forecasting.
One fascinating aspect of Predictive AI is its ability to analyze vast amounts of data in real-time, providing valuable insights that can drive strategic decision-making. For example, in the retail sector, Predictive AI algorithms can analyze customer behavior patterns to recommend personalized product offerings, leading to increased sales and customer satisfaction. Additionally, in the field of cybersecurity, Predictive AI can detect and prevent potential cyber threats by continuously monitoring network activities and identifying anomalies before they escalate into security breaches.
Furthermore, the application of Predictive AI extends to the field of manufacturing, where it can optimize production processes by predicting equipment failures before they occur, thus minimizing downtime and reducing maintenance costs. This proactive approach not only enhances operational efficiency but also improves overall productivity. As technology continues to advance, the potential of Predictive AI to transform various industries and drive innovation remains limitless.
Choosing Between Generative and Predictive AI

Choosing between Generative AI and Predictive AI is a crucial decision that can significantly impact the outcomes of your AI application. Generative AI focuses on creating new content, such as images, text, or music, based on patterns and examples it has learned during training. On the other hand, Predictive AI emphasizes making accurate predictions based on historical data and patterns. Understanding the nuances of each approach is essential in determining which one aligns best with your project goals.
When considering Generative AI, it’s important to evaluate the nature of the problem you are trying to solve. If your application requires generating new and innovative content, such as designing artwork or creating fictional stories, Generative AI can be a powerful tool. On the contrary, if your primary goal is to forecast trends, make data-driven decisions, or predict future outcomes based on historical data, Predictive AI might be the more suitable choice.
Factors to Consider
1. Nature of the problem: Determine whether you need novel content generation or accurate predictions.
2. Available data: Assess the availability and quality of data required for training and inference.
3. Application domain: Analyze the industry and use case to identify which approach aligns better with your goals.
4. Computational resources: Consider the computational power and infrastructure needed to support the training and deployment of your chosen AI model.
Making the Right Choice for Your Needs
Ultimately, making the right choice between Generative and Predictive AI requires a deep understanding of your project requirements and the capabilities of each approach. While Generative AI can spark creativity and innovation in fields like art and design, Predictive AI offers robust analytical capabilities for businesses seeking data-driven insights. Collaborating with AI experts and conducting thorough research can help you navigate the complexities of this decision-making process effectively.
The Future of AI: Generative and Predictive AI
As technology advances, the future of AI looks promising for both Generative AI and Predictive AI:
Artificial Intelligence (AI) has been a transformative force in various industries, and its evolution continues to shape the way we interact with technology. The convergence of Generative AI and Predictive AI is set to redefine the boundaries of what is possible, opening up new opportunities for innovation and growth.
Future Predictions for Generative AI
Generative AI is expected to continue innovating in creative fields, such as art, media, and entertainment. It will likely lead to the development of more realistic virtual environments, autonomous characters, and immersive experiences. Additionally, Generative AI holds the potential to create personalized learning experiences tailored to individual needs.
One exciting prospect for Generative AI is its application in the field of healthcare. By leveraging its creative capabilities, Generative AI can assist medical professionals in generating personalized treatment plans for patients based on their unique genetic makeup and medical history. This personalized approach has the potential to revolutionize the healthcare industry, leading to more effective treatments and improved patient outcomes.
Future Predictions for Predictive AI
Predictive AI will witness further advancements in precision and efficiency. As the availability of data increases, Predictive AI models will become more accurate and adaptable across a broader range of industries. This will enable businesses to make data-driven decisions with greater confidence and drive innovation.
Moreover, the integration of Predictive AI in the realm of cybersecurity is set to play a crucial role in safeguarding digital assets and protecting against cyber threats. By analyzing vast amounts of data in real-time, Predictive AI can proactively identify potential security breaches and vulnerabilities, allowing organizations to strengthen their defense mechanisms and mitigate risks effectively.
In Conclusion
The world of AI is vast and dynamic, with Generative AI and Predictive AI standing as two prominent pillars within it. Understanding the differences, advantages, and limitations of these approaches is crucial for harnessing their potential effectively. Whether you seek to create captivating art or make data-driven predictions, Generative AI and Predictive AI offer powerful tools that continue to shape our present and hold great promise for the future.